Calculating the practice benefit of universal bulk-billing - an updated model
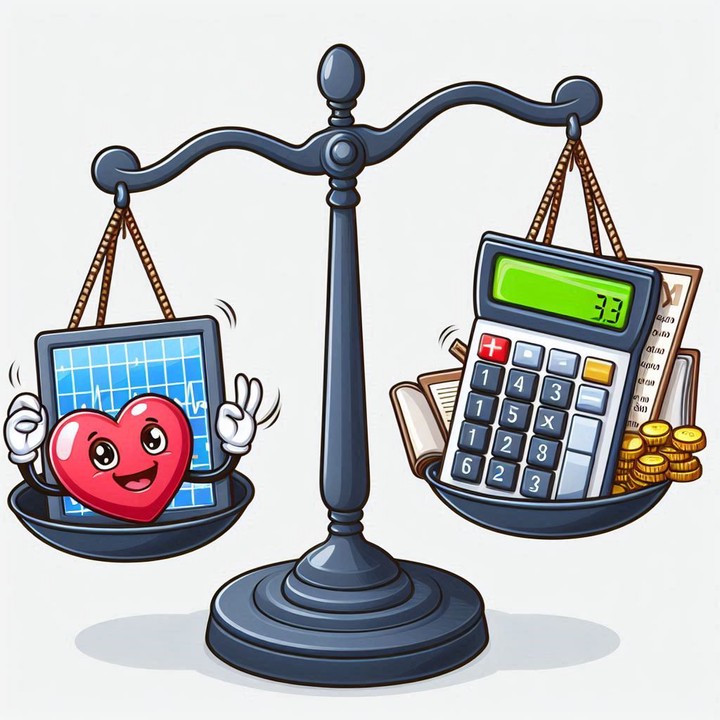
- 1 Universal bulk-billing incentive
- 2 Will the proposed universal bulk-billing incentives be financially worthwhile for general practices?
- 3 Modelling the benefits to individual practices
Updated from Calculating the practice benefit of universal bulk-billing. Updated model includes MBS Service Level A and, optionally, care plans (721/723/732).
Dashboard available on Amazon Web Services and ShinyApps.
Universal bulk-billing incentive
As part of an election promise, the Australian Government as of February 2025 released a proposal to increase the incentives for private general practices (sometimes known as ‘family medicine’ or ‘primary care’ practices) to bulk-bill patients. ‘Bulk-billing’ essentially allows a patient consultation with medical clinician to be ‘free’ (no out-of-pocket expense), if-and-only-if the clinician agrees to charge the patient no more than the government-set insurance price for the consultation.
The alternative to ‘bulk-billing’ is that the clinician charges the patient a price greater than the government-set insurance price, sometimes called private billing. In this case the patient pays the full price of the consultation and the government health insurance will repay the government-set insurance price to the patient.
The proposal to encourage general practices to ‘bulk-bill’ consisted of two parts:
- Broadening the current bulk-billing incentive for individual consultations/services to all patients.
- The government had provided bulk-billing incentives for general practitioners to bulk-bill patients previously deemed to benefit more from bulk-billing. The value of these incentives have been 7.15 to 21.35 dollars per service in urban areas.
- Currently the bulk-billing incentive payment for individual consultations is only available to people, usually with less income or assets, who are pensioners, recipients of a government-issued ‘concession card’ and children under the age of 16. The services which are bulk-billed and also qualify for the current bulk-billing incentive payment will be referred to as ‘concessionally bulk-billed’.
- This bulk-billing incentive is paid with each individual consult
- If the general practice agrees to bulk-bill all patients, i.e. universal bulk-billing, the government will pay the practice an additional 12.5% over and above the government-set price for each fee-for-service item. This incentive payment is sometimes called a ‘loading payment’.
- On 25th March 2025, the service items which needed to be bulk-billed, and attract the ‘loading’ payment were defined in the document Bulk Blling Practice Incentive Program: Eligible Services
- The definition of which service items need to be ‘bulk-billed’, and which service items will attract the 12.5% loading was not initially fully defined in the government-provided
fact sheet and
RACGP FAQ, although they did suggest that consultation items A/B/C/D, mental health care plan and other GP non-referred attendances will attract the 12.5% loading.
- For this reason, and simplicity, the initial description of this model is constrained to a small number of service types. Later, the model is expanded to all major service numbers, and can be easily expanded to all service types.
- The 12.5% loading is paid quarterly, together with the current quarterly practice incentive program (PIP) payments.
Will the proposed universal bulk-billing incentives be financially worthwhile for general practices?
The government has released case examples of practices which would all financially benefit from the universal bulk-billing incentives proposal. Not all real general practices have considered the incentives proposed to be attractive, however. The benefit to real practices may be more nuanced, depending on current private billing levels and current fees charged over the government-set price.
This model demonstrates the effect of pre-existing gap-fees, the proportion of patients which would normally be privately-billed and the inclusion of different non-referred general practice service items into the estimated benefit-loss of adopting the proposed bulk-bill incentive program.
Two examples are presented, followed by detailed description of the model and model variations.
Practice 1, limited item number inclusion
The first example practice currently concessionally bulk-bills (bulk-bills with the benefit of bulk-billing incentive payments) 50% of its patients. The benefit-loss calculation is based on just a few item numbers (‘A’, ‘B’, ‘C’, ‘D’ and ‘E’). The graph below demonstrates the effect on net-benefit of adopting the bulk-billing incentives, depending on the private ‘gap-fee’ charged by the practice (to those patients who were not bulk-billed or do not qualify for the current bulk-billing incentive payments) prior to adopting the universal-bulk-billing incentives. The light-blue vertical dotted line represents the net-benefit (-8.23 per service) if the practice charged an average gap-fee of $50.
Practice 2, low gap-fee, care-plan item numbers included
This example practice charges a low (mean) private gap-fee of $35, which applies to every person who is not bulk-billed or does not have access to current bulk-billing incentive rebates. The benefit-loss calculation is based on both standard numbers (‘A’, ‘B’, ‘C’, ‘D’ and ‘E’) and care-plan item numbers. This practice did not charge a gap-fee at all for care-plan item numbers, even for patients who did not have access to to current bulk-billing incentive rebates. The graph below demonstrates the effect on net-benefit of the adopting the bulk-billing incentives, depending on the pre-existing proportion of patients who were concessionally bulk-billed (with the benefit of current bulk-billing incentives) before adopting the universal-bulk-billing incentives.
The light-blue vertical dotted line represents the net-benefit (6.55 per service) if 80% of the practice population was concessionally bulk-billed.
Net benefit to practice heatmap table
An estimate of net benefit per service for a range of practices, assuming ‘average’ distribution of ABCDE, care plan, health assessment and mental health care plan items, depending on a range of mean gap fees charge to patients who were concessionally bulk-billed.
The heatmap table above helps demonstrate some of the definitions and assumptions in the presented model.
- In the ‘concessional bulk-billed 100%’ row in the table above is demonstrated the effect of the universal-bulk-billing incentive scheme on a practice whose practice population all have health care cards etc., and the practice bulk-bills them all. In this case, no additional patients will qualify for the individual service item bulk-bill incentives, but the practice qualifies for the 12.5% bulk-billing incentive ‘loading’ payment paid quarterly.
- All patients who do not qualify the current bulk-bill incentive rebates are ‘privately’ billed, even if the ‘gap fee’ is zero dollars (i.e. no gap fee charged above the government rebate for the service item). In the heatmap table above, this is shown most starkly by the item in the top-left corner, where the practice ‘privately’ bills 100% of patients, with a gap fee of zero dollars. In this case, the practice can agree to receive the 12.5% bulk-billing incentive loading payment paid quarterly AND all patients will now potentially qualify for the individual service item bulk-bill incentive.
- In practical terms, ‘gap fee’ in the heatmap table shown above is not the necessarily the ‘nominal’ gap fee for service items, but the mean gap fee charged when current individual service bulk-billing incentives do not apply. Which may be lower than the nominal gap fee, if lower gap fees are charged for certain services e.g. review appointments, care plans etc..
Following sections will describe how the models were created.
Modelling the benefits to individual practices
This model demonstrates the potential financial benefit (or loss) of taking up the universal bulk-billing proposal.
The proposition to test is:
$$ \small \text{Benefit} \ge \text{Loss} $$ Where Benefit is the benefit from universal bulk-billing and Loss is the revenue foregone from not charging a fee over and above the government-set price for the services.
Both the ‘Benefit’ and ‘Loss’ side of the equation can be broken down into several individual components.
$$ \small \text{Loss} = \text{ Private services } \times {\text{Mean Gap fee}} $$ Private services are the services which would have been privately billed - or did not have access to bulk-billing incentives - before a practice agrees to universal bulk-billing. The Mean Gap fee is the amount the clinician charges the patient over and above the government-set price for each service.
$$
\small
\begin{align}
\text{Benefit } = &\text{ Total fee-for-service billing } \times \text{universal bulk-billing incentive}\\\
&+ \text{Private Services } \times \text{ Individual service bulk-billing incentive}
\end{align}
$$
Services which would have been previously privately billed are billed at the lower government-set price for the service, but now attract an individual service bulk-billing incentive, at least partly offsetting the Loss from not charging a Gap fee.
$$
\small
\begin{align}
\text{Total fee-for-service billing } = &\text{ Billing for concessional bulk-billed services}\\\
&+ \text{Billing for private services}
\end{align}
$$
‘Bulk-billed’ services includes only those bulk-billed service (no ‘gap’ payment) where the patient currently qualifies for bulk-billing incentive payments. ‘Private’ services include patients where a ‘gap’ fee is charged, but also includes services where the patient was charged no ‘gap’ fee, but does not currently qualify for bulk-billing incentive payments (but will in the future plan).
If the practice agrees to universal bulk-billing, then all services which were previously ‘bulk-billed’ and previously ‘private-billed’ will benefit from the 12.5% universal bulk-billing incentive loading payment.
For more discussion about the mathematical relationship between benefit-per-service, relative benefit and mean fees, mean gap-fees and proportion of existing services which currently receive individual bulk-billing incentives, see ‘Further Mathematical exploration’
Services
All the ‘code’ below is fully modifiable, and can be changed to reflect individual practice circumstances! If you wish to ‘run’ the code, it is recommended that you ‘run’ the code blocks sequentially, from top to bottom. After you have ‘run’ a code block, you can modify the code and ‘run’ it again without running the previous code blocks.
If you ‘Run Code’ the code block below, a list of numbers in red will appear below the code block, showing the proportion of patients billed Medicare service items A, B, C, D and E.
A limitation of this model is that explicit details are not known regarding which service items are required to be bulk-billed under the proposal, and which services items will attract the universal bulk-billing incentive.
Released documents show that standard fee-for-service items, particularly items ‘A’, ‘B’, ‘C’ and ‘D’, will attract both bulk-billing incentives (individual service and universal bulk-billing incentive) and are required to be bulk-billed under the universal bulk-billing agreement. These are the most common service items to be charged in Australian general practice, and contribute the vast majority of practice revenue. There are other service item numbers, such as care-plan related service items (‘GPMP/721’, ‘TCA/723’ and ‘GPMP RV/732’), which contribute significantly to the practice income of many practices. Care-plan item numbers will be added to an example model described later.
Gap fees and bulk-billing incentive
Different practices and different clinicians charge different gap fees. The same clinician or practice may charge different gap fees for different services.
The government bulk-billing incentive for individual services varies by the location of the practice. The incentive typically increasing the less urban and more remote the location of the practice.
Calculating the benefit
We can now calculate the average benefit per patient if the practice adopts universal bulk-billing.
If you ‘Run Code’ the block below With the default settings of 50% of patients are concessionally bulk-billed and a mean gap fee of 50, the benefit-per-patient is shown as -8.23
, i.e. a loss of 8.23 compared to the current mean fee of 86.28
.
Note that ‘Run Code’ below will not work unless the previous code blocks have already been ‘Run’!!
Modifying the model for a different practice
What happens if we change some of the parameters? In this example, 80% of services are concessionally bulk-billed, the gap-fee for each privately-billed service item is $35 and the service items charged is biased towards the longer consult items.
If the mean gap fee is set to 35, the average consult length is longer and 80% of patients are concessionally bulk-billed, the practice can expect a mean benefit of 5.37
per service more than the current mean fee of 89.91
. Note that this practice has a higher current mean fee than the first practice, but longer consultation times would likely result in a lower fee-rate per hour.
Including care plan item numbers
The ‘Strengthening Medicare with more bulk billing’ information for GP providers and RACGP FAQ state that non-referred general practice service items such as care-plans and health assessments will also qualify for the new bulk-bill incentive payments. We can add these service items to our model. We will base this model on the second practice model.
Model limitation - It is expected that the service item numbers and descriptors for general practice care-plans (721, 723 and 732) will change late in 2025. This model assumes that the fee-for-service revenue for the practice through care-plans will remain similar after the change in care-plan services items occurs.
Compared to the
second practice model described above, adding care plan item numbers increases the mean benefit from 5.37
to 6.55
per service. The current mean fee has also risen, from 89.91
to 94.43
, reflecting the higher value of service items included.
Graphing the benefit
Different mean gap fees
We can look at the effect on benefit-loss by looking at a range of different mean gap fees. This graph is based on the first, original, example.
The above code chunk creates a plot like this, with a dashed red line at ‘zero’ benefit and a dotted blue line representing the current mean gap fee:
Different previous gap-billing proportion
Likewise, we can examine the effect on net benefit through a range of different proportion of patients being concessionally bulk-billed prior to adopting universal bulk-billing. This graph is based on the second example.
The above code block will produce a graph as below.
Heatmaps of benefit vs gap-fee and proportion privately-billed
We can draw a heatmap of benefit vs gap-fee and proportion privately-billed.
The data in these plots are based on ABCDE/care plan/health assessment and mental health care plan item proportions as found on Medicare statistics for 2024 i.e. the ‘mythical average’ general practice. As usual, you can modify the proportion yourself in the code below.
The code-block below also has code to generate 3D and interactive plots. Unfortunately, these 3D and interactive plots cannot be created ‘on-the-fly’ from the code-block on your web-browser, but can only be fully modified by copying into R/RStudio.
Below is an example 3D plot which is generated with the above code block. Note that the 3D plot below will not change if you manually change the values in the above code-block. To generate and modify this plot yourself, you will need to copy the code into RStudio/R and run the code yourself.
Code
library(tibble)
library(plotly)
library(ggplot2)
library(dplyr)
fee_names <- c("a", "b", "c", "d", "e")
service_fees <- array(
data = c(19.6, 42.85, 82.90, 122.15, 197.9),
dim = c(length(fee_names), 1),
dimnames = list(fee_names, "fee")
)
# the proportion which each item is billed
# raw figures are derived for calendar year 2024 Australia-wide
# http://medicarestatistics.humanservices.gov.au/statistics/mbs_item.jsp
service_proportion_raw <- array(
data = c(4037130, 81240293, 19899953, 1609147, 174664),
dim = c(length(fee_names), 1),
dimnames = list(fee_names, "n")
)
# calculate the proportions
# for simplicity, assume the same proportions for bulk-billed patients
# and privately-billed patients
service_proportion_bulk <- service_proportion_raw/sum(service_proportion_raw)
colnames(service_proportion_bulk) <- c("proportion")
service_proportion_private <- service_proportion_bulk
# Set the mean gap fee for the individual service items A, B, C, D and E
# for patients who were privately billed
# for simplicity, set to the same fee of $50
gap_fee <- array(
data = rep(50, length(fee_names)),
dim = c(length(fee_names), 1),
dimnames = list(fee_names, "gap")
)
# the proportion of patients who are concessional bulkbilled
# set to 50%
concessional_bulkbilled <- 0.5
# the bulk billing incentive, in dollars
# varies by location
# Modified Monash areas 1, 2, 3+4, 5, 6, 7
# we will index the above to 1, 2, 3, 4, 5 and 6 (merging areas 3+4)
# see https://www9.health.gov.au/mbs/search.cfm
n_monash <- 6 # six different Monash areas
individual_bulkbill_incentive <- array(
# there are two different individual bulk-billing incentive fees,
# 'standard' and 'triple'
data = c(
# standard bulk-billing incentive applies to service items like item A/'3'
# items 10990, 10991, 75855, 75856, 75857, 75858
c(7.15, 10.80, 11.45, 12.20, 12.85, 13.70),
# triple bulk-billing incentive applies to service items like item B, C and D
# items 75870, 75871, 75873, 75874, 75875, 75876
c(21.35, 32.50, 34.50, 36.65, 38.70, 41.10)
),
dim = c(n_monash, 2),
dimnames = list(c("1", "2", "3+4", "5", "6", "7"), c("single", "triple"))
)
# does the single- or triple- incentive apply to the fee item?
individual_bulkbill_incentive_by_fee <-
c("single", "triple", "triple", "triple", "triple")
# set the Monash area to the city
monash_area <- "1"
# universal bulk billing incentive 'loading' payment
# over the service fee
# set to 12.5%
universal_bulkbill_incentive <- 0.125
# show individual bulk-bill incentive applicable to defined Monash area
fee_bulkbill_incentive <- individual_bulkbill_incentive[monash_area, individual_bulkbill_incentive_by_fee]
# redefine dimensions of the array
dim(fee_bulkbill_incentive) <- c(length(fee_names), 1)
# change rownames to the fee names
rownames(fee_bulkbill_incentive) <- fee_names
fee_mean <- function(
# calculate the current mean fee
service_fees,
fee_names,
service_proportion_bulk,
service_proportion_private,
gap_fee,
concessional_bulkbilled,
individual_bulkbill_incentive,
individual_bulkbill_incentive_by_fee,
monash_area
) {
# calculate individual bulk-bill incentive applicable to defined Monash area
fee_bulkbill_incentive <-
individual_bulkbill_incentive[monash_area, individual_bulkbill_incentive_by_fee]
# re-define dimensions of the 'x' array
dim(fee_bulkbill_incentive) <- c(length(fee_names), 1)
# change row name to the fee names
rownames(fee_bulkbill_incentive) <- fee_names
(1 - concessional_bulkbilled) *
sum(service_fees * service_proportion_private) +
(concessional_bulkbilled) *
sum(service_fees * service_proportion_bulk) +
# the calculation above could be simplified, of course!, to
# sum(service_fees * service_proportion_private), but is kept
# separate to two different portions to clarify the calculation
# calculate (and add) the individual service bulk-billing incentive applied
# to patients currently bulk-billed
concessional_bulkbilled *
sum(service_proportion_private * fee_bulkbill_incentive) +
# calculate (and add) the private 'gap' fee
(1 - concessional_bulkbilled) * sum(service_proportion_private * gap_fee)
}
net_benefit <- function(
# calculate the average net benefit revenue
# to the practice per patient
# the variable names used in this function
# are the same as used in previous code chunks
# but will be 'local' to this function
service_fees,
fee_names,
service_proportion_bulk,
service_proportion_private,
gap_fee,
concessional_bulkbilled,
individual_bulkbill_incentive,
individual_bulkbill_incentive_by_fee,
monash_area,
universal_bulkbill_incentive) {
# calculate individual bulk-bill incentive applicable to defined Monash area
fee_bulkbill_incentive <- individual_bulkbill_incentive[monash_area, individual_bulkbill_incentive_by_fee]
# re-define dimensions of the 'x' array
dim(fee_bulkbill_incentive) <- c(length(fee_names), 1)
# change row name to the fee names
rownames(fee_bulkbill_incentive) <- fee_names
# calculate the universal bulk-bill incentive
# for patients formerly privately-billed
(1 - concessional_bulkbilled) *
sum(service_fees * service_proportion_private) *
universal_bulkbill_incentive +
# calculate (and add) benefit for patients already bulk-billed
concessional_bulkbilled *
sum(service_fees * service_proportion_bulk) *
universal_bulkbill_incentive +
# calculate (and add) the individual service bulk-billing incentive applied
# to patients formerly privately-billed
(1 - concessional_bulkbilled) * sum(service_proportion_private * fee_bulkbill_incentive) -
# calculate (and subtract) the loss from not charging a private 'gap' fee
(1 - concessional_bulkbilled) * sum(service_proportion_private * gap_fee)
}
# mean gap fees from $0 to $60
gap_fee_range <- seq(from = 0, to = 70, by = 1)
# privately billed range from 0 to 100%
concessional_bulkbilled_range <- seq(from = 0, to = 100, by = 1)
# this model is based on the first practice model described above
# together with care plan item numbers
# add new service fee descriptions
new_fee_names <- c(
fee_names,
# append chronic disease item numbers (721, 723, 732)
# health assessment item numbers (701 -- 715)
# and mental health care plan item numbers (2700 -- 271)
c("GPMP", "TCA", "CDM RV",
"HA701", "HA703", "HA705", "HA707", "HA715",
"MHCP2700", "MHCP2701", "MHCP2715", "MHCP2717", "MHCP RV")
)
new_service_fees <- array(
# append fees for 721, 723 and 732
data = c(service_fees,
164.35, 130.25, 82.10,
67.60, 157.10, 216.80, 306.25, 241.85,
81.70, 120.25, 103.70, 152.80, 81.70
),
dim = c(length(new_fee_names), 1),
dimnames = list(new_fee_names, "fee")
)
new_individual_bulkbill_incentive_by_fee <- c(
individual_bulkbill_incentive_by_fee,
rep("single", 3+5+5)
)
# and new service proportions
# from http://medicarestatistics.humanservices.gov.au/statistics/mbs_item.jsp (2024)
new_practice_service_raw <-
# A, B, C, D, E service items
# and GPMP/TCA/reviews (CDM), health assessment and mental health plan items
c(4037130, 81240293, 19899953, 1609147, 174664,
3357364, 2954994, 4319542,
21383, 165878, 273690, 463237, 263931,
97733, 31550, 904320, 211540, 504901)
new_practice_service_proportions <-
new_practice_service_raw/sum(new_practice_service_raw)
benefit_loss_practice_standard <- tibble(
gap = numeric(),
concessional_bulkbilled = numeric(),
current_fee_mean = numeric(),
benefit = numeric(),
benefit_rel = numeric(),
)
for (x in gap_fee_range) {
for (y in concessional_bulkbilled_range) {
new_gap_fee <- c(
rep(x, 5),
rep(x, length((new_fee_names))-5)
# mean gap fee charged for ABCDE, $0 for all others
)
current_fee_mean <- fee_mean(
service_fees = new_service_fees,
fee_names = new_fee_names,
service_proportion_bulk = new_practice_service_proportions,
service_proportion_private = new_practice_service_proportions,
gap_fee = new_gap_fee,
# set concessionally bulk-billed proportion to 'y'
# divide by 100 to convert from
# percentage to proportion
concessional_bulkbilled = y/100,
individual_bulkbill_incentive,
individual_bulkbill_incentive_by_fee = new_individual_bulkbill_incentive_by_fee,
monash_area
)
benefit <- net_benefit(
service_fees = new_service_fees,
fee_names = new_fee_names,
service_proportion_bulk = new_practice_service_proportions,
service_proportion_private = new_practice_service_proportions,
gap_fee = new_gap_fee,
# set concessional bulk-billed proportion to 'y'
# divide by 100 to convert from
# percentage to proportion
concessional_bulkbilled = y/100,
individual_bulkbill_incentive,
individual_bulkbill_incentive_by_fee = new_individual_bulkbill_incentive_by_fee,
monash_area,
universal_bulkbill_incentive
)
benefit_loss_practice_standard <- add_row(
benefit_loss_practice_standard,
gap = x, concessional_bulkbilled = y,
current_fee_mean = current_fee_mean,
benefit = benefit,
benefit_rel = benefit/current_fee_mean
)
}
}
# this plot cannot be shown in the web-browser using this code block!
# you will need to copy this code (and all the pre-requisites)
# into a 'R/RStudio' and run it yourself :)
fig_plotly_3d <- plot_ly(
data = benefit_loss_practice_standard,
x = ~gap, y = ~concessional_bulkbilled, z = ~benefit,
text = ~benefit_rel * 100, customdata = ~current_fee_mean,
type = "scatter3d", mode = "markers",
hovertemplate = paste(
"Mean Gap fee: <b>$%{x}</b><br>",
"Concessional bulk-billed: <b>%{y}</b>%<br>",
"Current mean fee: <b>%{customdata:$.2f}</b><br>",
"Benefit: <b>%{z:$.2f}</b><br>",
"Revenue change: <b>%{text:.0f}</b>%",
"<extra></extra>"
),
marker = list(
color = ~benefit,
colorscale = "Rainbow",
showscale = TRUE,
line = list(width = 1, color = "DarkSlateGrey"))
) |>
layout(
title = "Benefit-loss according to mean gap fee and proportion concessional bulkbilled",
scene = list(
xaxis = list(title = list(text = "Mean Gap fee ($)")),
yaxis = list(
title = list(text = "Concessional bulk-billed (%)"),
autorange = "reversed"
),
zaxis = list(title = list(text = "Net benefit per service ($)"))
),
margin = list(r = 150)
# coloraxis = list(colorbar = list(x = 0.6))
# move the color legend to the left a little
)
# this plot cannot be shown in the web-browser using this code block!
# you will need to copy this code (and all the pre-requisites)
# into a 'R/RStudio' and run it yourself :)
fig_ggplotly <- ggplot(
data = benefit_loss_practice_standard,
aes(x = gap, y = concessional_bulkbilled))+
geom_point(
aes(fill = benefit),
size = 4, shape = 22, stroke = 0
) +
scale_fill_viridis_c(option = "turbo", direction = -1)
# to look at this plot, either 'fig_ggplotly' in R/RStudio or
# 'ggplotly(fig_ggplotly)'
fig_plotly_3d # show the 3D Plotly
The following interactive plot is based on the second practice model, with relatively long consults and low mean gap fees and did not charge gap-fees for care plans or health assessments for any patients. Again, the plot below cannot be changed ‘in-browser’, but you can recreate this plot if you copy the code into R/RStudio.
Code
new_practice_service_raw_2 <-
# A, B, C, D, E service items
# and GPMP/TCA/reviews (CDM), health assessment and mental health plan items
c(950, 3956, 4171, 1146, 75,
416, 402, 255,
0, 13, 101, 45, 1,
7, 5, 64, 31, 41)
new_practice_service_proportions_2 <-
new_practice_service_raw_2/sum(new_practice_service_raw_2)
benefit_loss <- tibble(
gap = numeric(),
concessional_bulkbilled = numeric(),
current_fee_mean = numeric(),
benefit = numeric(),
benefit_rel = character()
)
for (x in gap_fee_range) {
for (y in concessional_bulkbilled_range) {
new_gap_fee <- c(
rep(x, length((new_fee_names)))
# the same mean gap fee charged for every item, including GPMP/TCA/GPMPRV items
# a simplified approach would use the 'average' mean gap fee charged
# for all relevant items
)
current_fee_mean <- fee_mean(
service_fees = new_service_fees,
fee_names = new_fee_names,
# set service proportions to longer consults
service_proportion_bulk = new_practice_service_proportions_2,
service_proportion_private = new_practice_service_proportions_2,
gap_fee = new_gap_fee,
# set concessional bulk-billed proportion to 'y'
# divide by 100 to convert from
# percentage to proportion
concessional_bulkbilled = y/100,
individual_bulkbill_incentive,
individual_bulkbill_incentive_by_fee = new_individual_bulkbill_incentive_by_fee,
monash_area
)
benefit <- net_benefit(
service_fees = new_service_fees,
fee_names = new_fee_names,
# set service proportions to longer consults
service_proportion_bulk = new_practice_service_proportions_2,
service_proportion_private = new_practice_service_proportions_2,
gap_fee = new_gap_fee,
# set concessional bulk-billed proportion to 'y'
# divide by 100 to convert from
# percentage to proportion
concessional_bulkbilled = y/100,
individual_bulkbill_incentive,
individual_bulkbill_incentive_by_fee = new_individual_bulkbill_incentive_by_fee,
monash_area,
universal_bulkbill_incentive
)
benefit_loss <- add_row(
benefit_loss,
gap = x, concessional_bulkbilled = y,
current_fee_mean = current_fee_mean,
benefit = benefit,
benefit_rel = formatC((benefit / current_fee_mean * 100), digits = 0, format = "f")
)
}
}
# this plot cannot be shown in the web-browser using this code block!
# you will need to copy this code (and all the pre-requisites)
# into a 'R/RStudio' and run it yourself :)
fig_ggplotly <- ggplot(
data = benefit_loss,
aes(
x = gap, y = concessional_bulkbilled,
text = sprintf(
paste(
"Gap: $%d<br>Concessional bulk-billed: %d%%<br>Current fee mean: $%.2f<br>",
"Benefit: $%.2f<br>Revenue change: %s%%", sep = ""
),
gap, concessional_bulkbilled, current_fee_mean, benefit, benefit_rel
)
)) +
geom_point(
aes(fill = benefit),
size = 4, shape = 22, stroke = 0
) +
labs(
x = "Mean Gap fee ($)",
y = "Concessional bulk-billed (%)",
fill = "Benefit ($)"
) +
scale_fill_viridis_c(option = "turbo", direction = -1)
# to look at this plot, either 'fig_ggplotly' in R/RStudio or
# 'ggplotly(fig_ggplotly)'
ggplotly(fig_ggplotly, tooltip = "text") |> # show the ggplotly
layout(margin = list(r = 150))
The interactive benefit-loss heatmap above is reproduced in a more conventional table format below.
Calculating relative (percentage) change in revenue
Some of the interactive plots above include the estimated proportional change in revenue (mean fee) as the result of adopting universal bulk-billing. This is calculated from
$$ \small \frac{\text{Net benefit (profit or loss)}}{\text{Current mean fee}} $$
The following table is based on the second practice model.
Following is a 3D plot of percentage change in revenue as the result of adopting universal bulk-billing for the ‘average’ general practice, according to pre-existing gap-fee and proportion of services which are privately-billed.
Code
# 3D plot of 'standard' Australian practice
# percentage change in revenue vs pre-existing mean gap fee and 'private'-billing proportion
# the data underlying this plot cannot be dynamically changed in the web-browser
# you will need to copy this code (and all the pre-requisites)
# into 'R/RStudio' and run it yourself :)
fig_plotly_3d <- plot_ly(
data = benefit_loss_practice_standard,
x = ~gap, y = ~concessional_bulkbilled, z = ~benefit_rel * 100,
text = ~benefit, customdata = ~current_fee_mean,
type = "scatter3d", mode = "markers",
hovertemplate = paste(
"Mean Gap fee: <b>$%{x}</b><br>",
"Concessional bulk-billed: <b>%{y}</b>%<br>",
"Current mean fee: <b>%{customdata:$.2f}</b><br>",
"Benefit: <b>%{text:$.2f}</b><br>",
"Revenue change: <b>%{z:.0f}</b>%",
"<extra></extra>"
),
marker = list(
color = ~benefit,
colorscale = "Rainbow",
showscale = TRUE,
line = list(width = 1, color = "DarkSlateGrey"))
) |>
layout(
title = "Revenue change (%) according to mean gap fee and proportion privately-billed",
scene = list(
xaxis = list(title = list(text = "Mean Gap fee ($)")),
yaxis = list(
title = list(text = "Concessional bulk-billed (%)"),
autorange = "reversed"
),
zaxis = list(title = list(text = "Revenue change (%)"))
),
margin = list(r = 150)
# coloraxis = list(colorbar = list(x = 0.6))
# move the color legend to the left a little
)
fig_plotly_3d
Reproducing the universal bulk-billing fact sheet table
(Supplement)
We can reproduce part of the table shown in the government-provided fact sheet representing the total payments received (both individual bulk-billing incentive and universal bulk-billing incentive) for each service for those practices which agree to universally bulk-bill. The original table is shown below:
Note that ‘Run Code’ below will not work unless the previous code blocks have already been ‘Run’!!
The above code block output is shown below, matching the table in the fact sheet.
Further mathematical exploration
Mean service revenue before adopting universal bulk-billing $r_0$
$$
\small
\begin{aligned}
r_0 &= bx + bi + (1-b)x + (1-b)g\\\
&= x + g + b(i-g) &(1)\\\
\end{aligned}
$$
$$
\small
\begin{aligned}
\text{where }b &= \text{proportion of patients bulk-billed concessionally (0-1)}\\\
x &= \text{mean service fee}\\\
i &= \text{mean individual service bulk-billing incentive}\\\
g &= \text{mean gap-fee when patient not bulk-billed with incentive fee}\\\
\end{aligned}
$$
Mean service revenue after adopting universal bulk-billing $r_1$
$$
\small
\begin{aligned}
r_1 &= ux + i &(2)\\\
\end{aligned}
$$
$$
\small
\begin{aligned}
\text{where } u &= \text{rebates (100%) plus quarterly loading payment (12.5%)} = 1.125\\\
x &= \text{ mean service fee}\\\
i &= \text{ mean individual service bulk-billing incentive}\\\
\end{aligned}
$$
Mean benefit per service ($) of adoping universal bulk-billing
Mean benefit per service of adopting universal bulk-billing $p$
$$
\small
\begin{aligned}
p &= r_1 - r_0 \\\
&= x(u-1) - (g-i)(1-b)&(3)\\\
\end{aligned}
$$
Implications of equation for $p$ (Equation 3):
- The greater the mean service fee $x$ the greater the mean benefit (per-service) $p$ of adopting universal bulk-billing i.e. $p\propto x$
- $x$ may increase if longer consult items (‘C’ vs ‘B’) are used, or if higher-rebate items are more frequently used e.g. care plans and health assessment.
- The greater the mean gap-fee $g$ increases over and above the mean individual service bulk-billing incentive $i$, the less the mean benefit (per-service) $p$ of adopting universal bulk billing i.e. $p\propto-(g-i)$
- The mean gap-fee $g$ is less than the ‘nominal’ gap-fee if the gap-fee is not charged for all relevant services e.g. care-plans, health assessments, reviews.
- For example, if a nominal gap-fee of 35 dollars is charged for only 50% of services which don’t attract a current bulk-billing incentive, then the mean-gap fee $g$ will be 17.5 dollars.
- The mean individual service bulk-billing incentive $i$ can change with service billing patterns, as some services attract a ‘single’ incentive of 7.15 or a ‘triple’ incentive of 21.35 (in Monash Area 1).
- For example, if a clinic charges more care plans (which attract a single incentive) and less BCDE services (which attract a triple incentive), then $i$ will be reduced.
- For the ‘average’ general practice, according to MBS 2024 figures, $i$ is 19.28. For the practice which does - relatively - more care plans and described as ‘practice 2’ above, $i$ is 18.52.
- As pre-existing bulk-billing (with existing bulk-billing incentives) increases $(b \to 1)$, the less influence the difference between mean gap-fee $g$ and mean bulk-billing incentive fee $i$ have over mean benefit per-service $p$ i.e. $\displaystyle{\lim_{b\to1} p(b) = x(u-1)}$. Conversely, as $b \to 0$, the greater the influence of $(g-i)$ on $p$.
Relative benefit of adopting universal bulk-billing
The relative benefit of adopting universal bulk-billing $q$
$$
\small
\begin{aligned}
q &= \frac{p}{r_0}\\\
&= \frac{x(u-1) + (i-g)(1-b)}{x + g + b(i-g)} &(4)\\\
&= \frac{x(u-1) - (g-i)(1-b)}{x + (g-i)(1-b) + i} &(5)\\\
\end{aligned}
$$
$$
\small
\begin{aligned}
\text{where } x &= \text{mean service fee}\\\
u &= \text{rebates (100%) plus quarterly loading payment (12.5%)} = 1.125\\\
g &= \text{mean gap-fee when patient not bulk-billed with incentive fee}\\\
i &= \text{mean individual service bulk-billing incentive}\\\
b &= \text{proportion of patients bulk-billed concessionally (0-1)}\\\
\end{aligned}
$$
Implications of equation for $q$ (Equation 5)
- As the mean service fee $x$ increases $(x \to \infty)$ then $q \to (u-1)$ i.e. $\displaystyle{\lim_{x \to \infty} q(x) = 0.125}$
- $q$ is quite sensitive to $(g-i)(1-b)$, which appears in both the numerator and denominator, but with opposite signs.
- $q$ is sensitive to the difference between the mean gap-fee $g$ and the mean individual bulk-bill incentive $i$ i.e. $q\propto-(g-i)$
- As the gap-fee increases $g\to \infty$ then $\displaystyle{\lim_{g\to\infty}q=\frac{-g}{+g}}=-1$.
- As the proportion of bulk-billed with pre-existing incentives increases $b\to1$ then $\displaystyle{\lim_{b\to1}q(b) = \frac{x(u-1)}{x+i}}$
- As the proportion of bulk-billed with pre-existing incentives falls $b\to0$ then $\displaystyle{\lim_{b\to0}q(b) = \frac{x(u-1)-(g-i)}{x+g}}$
Technical credits
Thanks to the quarto-webr extension, Claudiu-Cristian’s post on using webR in hugo websites and WebR itself for the ability to run modifiable R models on ‘static’ web-pages! Thanks to Dr Michael Tam for suggesting adding 3D plots and colour!
External commentary and other models
An article on the Medical Republic ‘Which of these four Medicare BB models is Right for You?' compares some of the available (and not yet available) models - including this one! - and the advantages and disadvantages of each.